From the Non-ergodicity in Physics to the Non-convexity in Optimization – How do They Manifest Themselves in the Variable Space? Implications and Applications
- 项目计划:
- 优配研究金
- 项目年份:
- 2023/2024
- 项目负责人:
- 杨志豪博士
- (科学与环境学系)
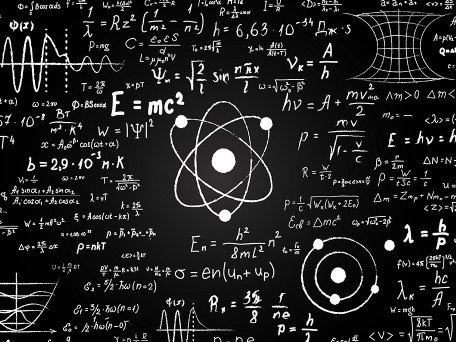
Indeed, rugged energy landscapes in spin glass share similar features with non-convex objective functions in non-convex optimization problems.
In physics, a system is non-ergodic if it fails to visit all equally probable equilibrium states even after a very long time. A representative non-ergodic model is spin glass. To explain its non-ergodicity, physicists utilize energy landscapes, which are high-dimensional functions representing the dependence of system energy on variable configurations. The non-ergodicity of spin glass is then associated with rugged energy landscapes, characterized by exponentially many local minima and high-energy barriers, thus trapping the system in a subset of all probable states. We seem to understand well how energy landscapes influence emergent behaviors of physical systems, but how landscape-features such as barriers and plateaus manifest themselves in the variable space is less understood. Interestingly, one can only control how a system moves across barriers and plateaus in energy landscapes through manipulating variables in the variable space, but an understanding of how to connect energy landscapes with variable space is missing. Indeed, rugged energy landscapes in spin glass share similar features with non-convex objective functions in non-convex optimization problems. This non-convexity may have a non-trivial origin instead of simply an explicit form of non-convex objective functions or constraints. For instance, deep neural networks (DNNs), which are universal function approximators, can represent both convex and non-convex functions by optimizing the same form of loss function subject to different datasets. The increasing use of DNNs on artificial intelligence applications is also a major reason for the rapid increase in the interest of non-convex optimization problems in recent years. Similar to spin glass, the causal link between variable space and cost/loss landscape in non-convex optimization problems is largely unknown. In the proposed project, we aim to
- Generate new understandings to link features in the spin space to features in the rugged energy landscapes of spin glass, to better understand non-ergodicity in physics;
- Transfer our new understanding to link the variable space and the cost/loss landscape of non-convex optimization problems, to better understand non-convexity in optimization;
- Derive new barrier-overcoming and plateau-overcoming mechanisms for both spin glass and non-convex optimization problems;
- Integrate the new mechanisms in blending existing optimization approaches in both areas, leading to a new optimization paradigm for both non-ergodic physics systems and non-convex optimization problems.
The project aims to contribute to both community of spin glass and non-convex optimization problems, and to non-convex optimization applications including deep learning and recommender systems.